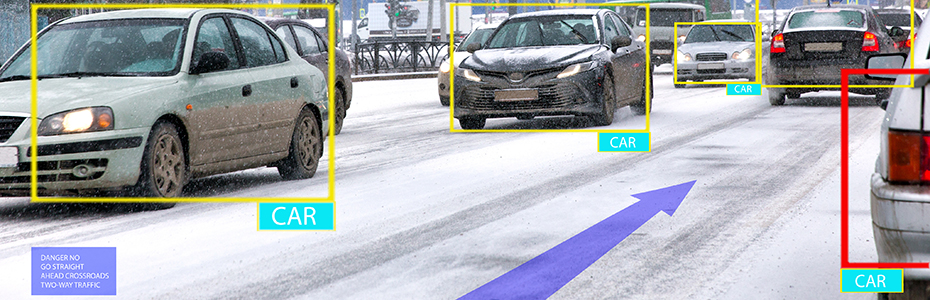
Together with the Fraunhofer institutes IKS, ITWM and IOSB, this Fraunhofer-internal project focuses on the development of an integrated framework for verifying “safeML”. The aim is to enable innovative machine learning methods to be deployed in safety-relevant applications. This will help manufacturers of autonomous, safety-critical cyber-physical systems, their suppliers, and the respective testing and approval institutions to bring ML-based systems to market that feature a comparable safety level to established software-based approaches.
Deep neural networks and other machine learning (ML) methods make it possible to model highly complex relationships in a data-driven manner, thus increasing the degree of autonomy in cyber-physical systems. However, concerns about the reliability and robustness of ML models, the limited explainability of decision making processes, and the lack of meaningful safety metrics have so far prevented their widespread deployment in safety-related applications. There are two main challenges:
1) ML for safety-critical applications in a limited field of use: Current ML methods do not yet achieve the low error rates required for safety-critical software. There is a lack of appropriate standards and development methods to demonstrate the residual risk and safety-related failure associated with ML components. An example of this is stationary applications, such as cobots.
2) ML for safety-critical applications without limitations: Compensatory measures on the system side and usage restrictions that have to be applied due to the error rates of ML functions result in prohibitive costs or limit the availability of the corresponding function. Because of this, ML methods need to be optimized in terms of domain-specific acceptance criteria and quantitatively proven. An automated, iterative approach is required to support modifications in function and the permissible range of use, as well as to lower development costs. An example of this is mobile applications, such as autonomous vehicles.
To ensure that the industrialization of ML processes can also achieve its enormous growth potential in the domains of autonomous vehicles, construction machinery and cobots in the future, the project focuses on the following innovations:
1) Demonstrating the general suitability of ML techniques to meet safety-critical requirements in the selected application domains.
2) Demonstrating how the likelihood of residual safety-related failures can be minimized by combining complementary design methods, analysis techniques, simulation-based tests, and runtime mechanisms.
3) Demonstrating that the same level of confidence can be attained in ML models in cyber-physical systems as with today's safety-critical software.