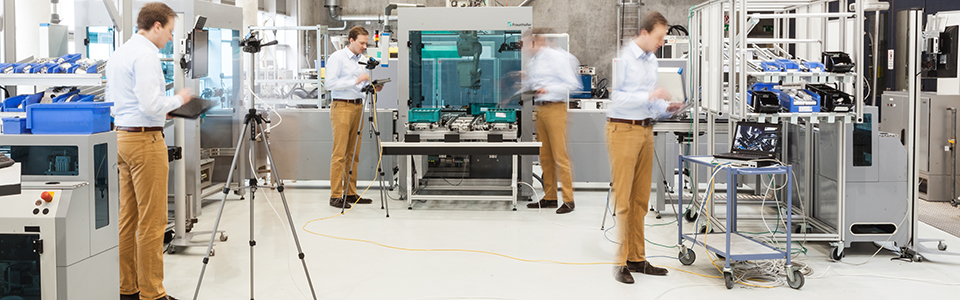
At the automotive supplier Adient, Fraunhofer IPA analyzed the causes of downtimes and rejects occurring with a fast-cycle, automated manufacturing system for producing fittings to adjust the position of vehicle seats. The data-driven analysis resulted in optimization measures that have sustainably increased the productivity of the manufacturing system.
The manufacturing system has a high number of interconnected production steps with a cycle time of three seconds, making it very difficult to identify the causes of downtimes and rejects. Thanks to "Smart System Analysis and Optimization", the causes of even complex errors could be pinpointed accurately. As part of the pilot project at Adient, the scientists acquired signal data from machine controllers (Siemens S7-400) and additionally integrated sensors (Cognex camera inspection systems, temperature sensors) as well as cameras for object recognition. Using specially-developed algorithms to analyze causes and effects, they were able to derive an OEE (Overall Equipment Effectiveness) optimization potential of 8.6 percent within a very short time and verify the appropriate measures with the help of simulation.
In the course of the project, initial "quick wins" amounting to 3.5% have already been implemented. For instance, ways of adjusting control parameters were identified, which would not have been discovered in the thousands of control variables and sequence dependencies without the data-driven approach. In particular, the method enabled complex error patterns resulting from mutual interactions between several successive production steps to be identified. The design of buffers as well as the strategy for removing workpieces for rework or as rejects could also be quantified and optimized in a second step.
Adient now wants to adopt this method for larger manufacturing systems involving more than 50 production and assembly processes. This will allow the company to analyze the systems continuously and acquire data on current complex error patterns in real time. The scientists at IPA are already working on a scalable version of their tool.