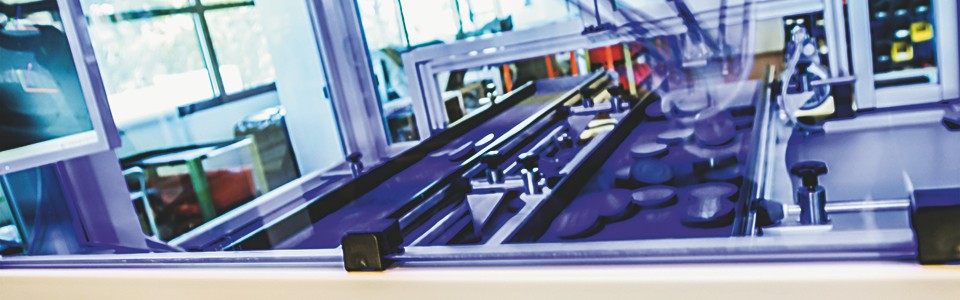
In the manufacturing sector, there is often a lack of flexible automation solutions, especially in component feeding. Workpieces are often stored in disorganized fashion in crates or pallet cages and then separated by spiral conveyors and conveyor belts with complex mechanics specially adapted to the parts. This makes such automated feeders inflexible in the face of component changes, and any adjustments which need to be made to the feeding technology are time-consuming and expensive. Alternatively, robots can be used to pick up the disorderly parts directly out of the crates or off the conveyor belt. However, precise 3D detection of the objects or their position is essential.
To this end, various 3D recognition methods have been developed. Based on CAD data or even entirely without a component model, these methods recognize the component directly in the measurement data and accurately determine its position in space.
Recognizing 3D object positions in 3D measuring point clouds without a CAD model is based on best-fit methods for regular geometric elements such as planes, cylinders, cones, spheres or tori. This method was chosen because many workpieces are cylindrical or conical in shape. However, the objects to be recognized do not have to be cylinders or cones - significantly more complex workpieces can also be recognized.
3D object recognition solutions based on CAD models have also been developed. These are used, in particular, if the component to be detected does not feature any dominant regular geometric elements, but consists mainly of free-form surfaces or has a complex geometry.
Until now, complete CAD models could not be fitted into the measurement data because calculation times were too long. The new techniques for best-fitting STL or CAD models into 3D measurement point clouds can, depending on the required accuracy of the result, use different calculation methods during the optimization and thus greatly accelerate the calculation.